Computer Algorithm Seen to Predict Lymphoma in Primary Sjögren’s with High Accuracy
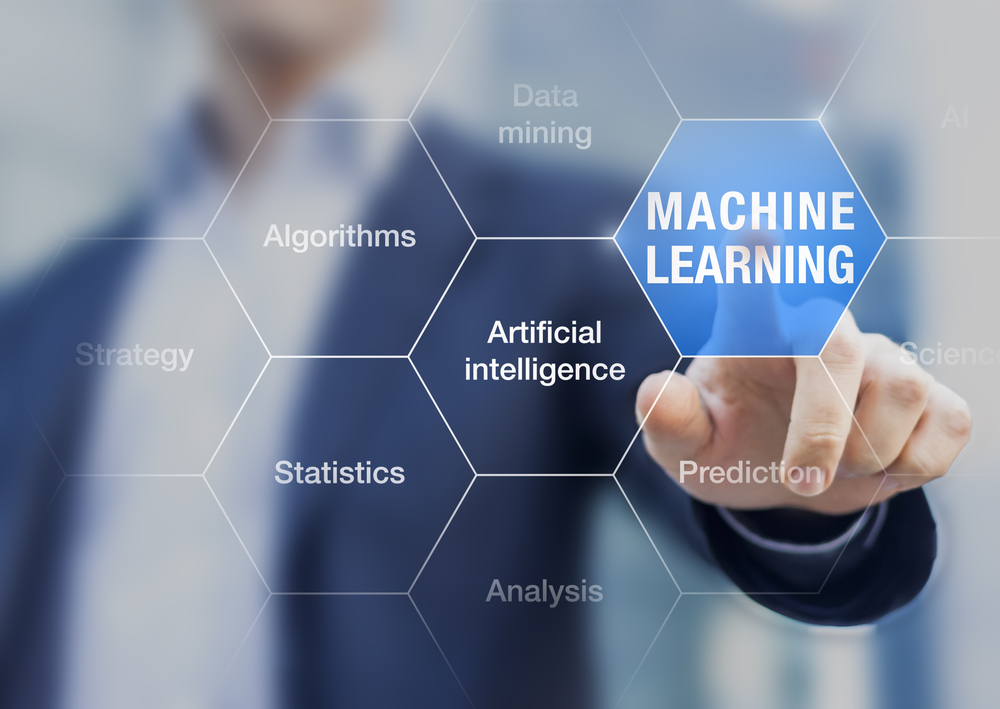
A new machine learning algorithm may help identify people with primary Sjögren’s syndrome who are at an increased risk of developing non-Hodgkin’s lymphoma (NHL), a type of blood cancer, findings from a new study suggest.
Using risk features such as enlarged salivary glands, counts of certain types of immune cells at diagnosis, and the presence of ‘blood spots’ called purpura, the algorithm correctly identified about 83% of samples as being either controls or lymphoma patients.
The findings also indicate a good prognosis for Sjögren’s patients who develop non-Hodgkin’s lymphoma, with an estimated 10-year survival rate of at least 80%.
The research was presented at the 2019 American College of Rheumatology/The Association of Rheumatology Professionals (ACR/ARP) Annual Meeting in a poster titled, “Data Driven Prediction Lymphoma Model and 10-year Overall Survival Rates of a Large Harmonized Cohort of Patients with Primary Sjögren’s Syndrome Associated Lymphomas.”
Machine learning involves feeding data into a computer, and giving the computer instructions to extract insights from that data — for example, sorting between healthy people and those with a condition. The computer then develops algorithms that can carry out that function (the ‘learning’ part of machine learning) in new data, effectively classifying samples as controls or diseased.
In this instance, researchers fed into computers clinical data covering 162 people with primary Sjögren’s syndrome who developed a non-Hodgkin’s lymphoma, as well as data for 278 Sjögren’s patients who had no history of lymphoma. The two patient groups were similar with regard to age, sex, and disease duration.
The computer was instructed to find ways to sort those with lymphoma from those without — ultimately helping researchers identify risk factors predicting lymphomas in these patients.
The algorithm that was ultimately generated was then tested by feeding it data it had never seen before, and seeing how well it did. The sensitivity of the algorithm — that is, the percentage of lymphoma patients who were accurately identified as such — was 75%. Its positive predictive value — the proportion of lymphoma patients accurately identified as such over the total lymphoma predictions — was 83%.
Looked at another way, the average area under the curve (AUC) of the algorithm was calculated at 0.90. In this instance, AUC is basically a statistical measurement of how well two groups can be separated, with values closer to 1 indicating better distinguishing power.
Closer inspection of the computer-generated algorithm revealed some of the most influential features used in this differentiation. That is, the risk factors for lymphoma in these people.
These included salivary gland enlargement lasting over two months, enlargement of the salivary and/or tear glands at Sjögren’s onset, count of neutrophils (a type of immune cell) at diagnosis, the presence of palpable purpura, and hypergammaglobulinemia (abnormally high levels of antibodies in the blood). People with Sjögren’s who have these risk factors may benefit from closer monitoring for lymphoma.
The researchers also estimated the 10-year survival rates for Sjögren’s patients diagnosed with a lymphoma.
The overall 10-year survival rate was estimated at 87%, with some variation based on specific lymphoma type: 88.2% for mucosal associated lymphoid tissue lymphomas (MALTL), and 80% for diffuse large B-cell lymphomas (DLBCL).
From this, the researchers concluded that, “[Sjögren’s syndrome] patients with NHLs display a favorable prognosis, especially those with MALTL.”